Dave*, Vedant; Lygerakis*, Fotios; Rueckert, Elmar Multimodal Visual-Tactile Representation Learning through Self-Supervised Contrastive Pre-Training Proceedings Article In: IEEE International Conference on Robotics and Automation (ICRA 2024)., 2024, (* equal contribution). @inproceedings{Dave2024b,
title = {Multimodal Visual-Tactile Representation Learning through Self-Supervised Contrastive Pre-Training},
author = {Vedant Dave* and Fotios Lygerakis* and Elmar Rueckert},
url = {https://cloud.cps.unileoben.ac.at/index.php/s/Nw9TprfdDoLgr8e},
year = {2024},
date = {2024-01-28},
urldate = {2024-01-28},
booktitle = {IEEE International Conference on Robotics and Automation (ICRA 2024).},
note = {* equal contribution},
keywords = {deep learning, Tactile Sensing, University of Leoben},
pubstate = {published},
tppubtype = {inproceedings}
}
| 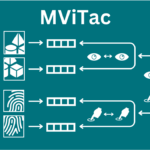 |
Lygerakis, Fotios; Rueckert, Elmar CR-VAE: Contrastive Regularization on Variational Autoencoders for Preventing Posterior Collapse Proceedings Article In: Asian Conference of Artificial Intelligence Technology (ACAIT)., 2023. @inproceedings{Lygerakis2023,
title = {CR-VAE: Contrastive Regularization on Variational Autoencoders for Preventing Posterior Collapse},
author = {Fotios Lygerakis and Elmar Rueckert},
url = {https://cloud.cps.unileoben.ac.at/index.php/s/fNNRnzJFPrtGQM2},
year = {2023},
date = {2023-08-16},
urldate = {2023-08-16},
booktitle = {Asian Conference of Artificial Intelligence Technology (ACAIT).},
keywords = {computer vision, deep learning, University of Leoben},
pubstate = {published},
tppubtype = {inproceedings}
}
| 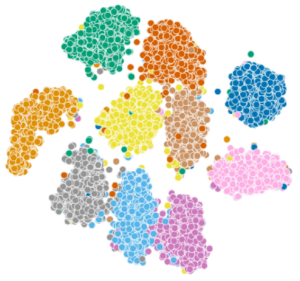 |
Xue, Honghu; Hein, Benedikt; Bakr, Mohamed; Schildbach, Georg; Abel, Bengt; Rueckert, Elmar Using Deep Reinforcement Learning with Automatic Curriculum Learning for Mapless Navigation in Intralogistics Journal Article In: Applied Sciences (MDPI), Special Issue on Intelligent Robotics, 2022. @article{Xue2022,
title = {Using Deep Reinforcement Learning with Automatic Curriculum Learning for Mapless Navigation in Intralogistics},
author = {Honghu Xue and Benedikt Hein and Mohamed Bakr and Georg Schildbach and Bengt Abel and Elmar Rueckert},
url = {https://cloud.cps.unileoben.ac.at/index.php/s/yddDZ7z9oqxenCi},
doi = {10.3390/app12063153},
year = {2022},
date = {2022-01-31},
urldate = {2022-01-31},
journal = {Applied Sciences (MDPI), Special Issue on Intelligent Robotics},
abstract = {We propose a deep reinforcement learning approach for solving a mapless navigation problem in warehouse scenarios. The automatic guided vehicle is equipped with LiDAR and frontal RGB sensors and learns to reach underneath the target dolly. The challenges reside in the sparseness of positive samples for learning, multi-modal sensor perception with partial observability, the demand for accurate steering maneuvers together with long training cycles. To address these points, we proposed NavACL-Q as an automatic curriculum learning together with distributed soft actor-critic. The performance of the learning algorithm is evaluated exhaustively in a different warehouse environment to check both robustness and generalizability of the learned policy. Results in NVIDIA Isaac Sim demonstrates that our trained agent significantly outperforms the map-based navigation pipeline provided by NVIDIA Isaac Sim in terms of higher agent-goal distances and relative orientations. The ablation studies also confirmed that NavACL-Q greatly facilitates the whole learning process and a pre-trained feature extractor manifestly boosts the training speed.},
keywords = {autonomous navigation, computer vision, deep learning},
pubstate = {published},
tppubtype = {article}
}
We propose a deep reinforcement learning approach for solving a mapless navigation problem in warehouse scenarios. The automatic guided vehicle is equipped with LiDAR and frontal RGB sensors and learns to reach underneath the target dolly. The challenges reside in the sparseness of positive samples for learning, multi-modal sensor perception with partial observability, the demand for accurate steering maneuvers together with long training cycles. To address these points, we proposed NavACL-Q as an automatic curriculum learning together with distributed soft actor-critic. The performance of the learning algorithm is evaluated exhaustively in a different warehouse environment to check both robustness and generalizability of the learned policy. Results in NVIDIA Isaac Sim demonstrates that our trained agent significantly outperforms the map-based navigation pipeline provided by NVIDIA Isaac Sim in terms of higher agent-goal distances and relative orientations. The ablation studies also confirmed that NavACL-Q greatly facilitates the whole learning process and a pre-trained feature extractor manifestly boosts the training speed. | 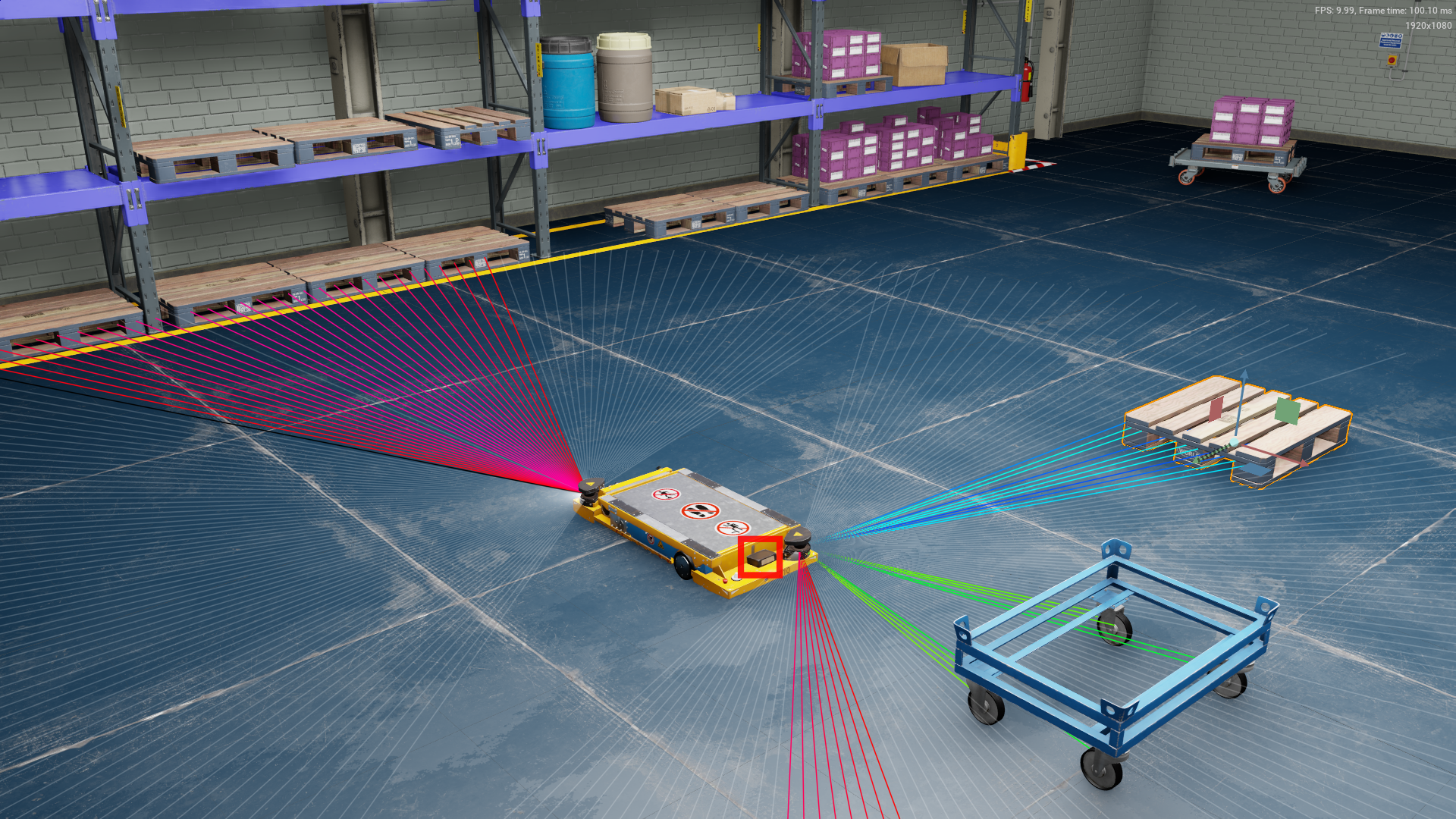 |